Artificial Intelligence (AI) is developing at an astonishing pace. Just last year, generative AI tools like ChatGPT and Gemini (formerly Bard) dominated tech and business conversations about the future. Now, there's even an AI politician named Steve running for a Parliamentary seat in the UK.
The application of AI could change the world even more than ENIAC, the first computer ever built. This 30-meter-long and three-meter-high machine, capable of performing complex calculations in seconds that took humans hours, now seems closer to a simple abacus compared to contemporary algorithms. AI surpasses this by not only being faster but also capable of translating, prioritizing, interpreting, and analyzing vast amounts of information.
Industries and businesses of all kinds are beginning to integrate AI into their daily operations. However, as with any innovation, we have yet to fully realize its potential.
Today, we want to discuss an essential function that all growing businesses need to incorporate into their operations and strategies: analytics. Effective analytics require integrating as many variables as possible to better understand flaws, assets, competition, production, and profit. AI analytics can simplify and enhance this process, and we want to show you how.
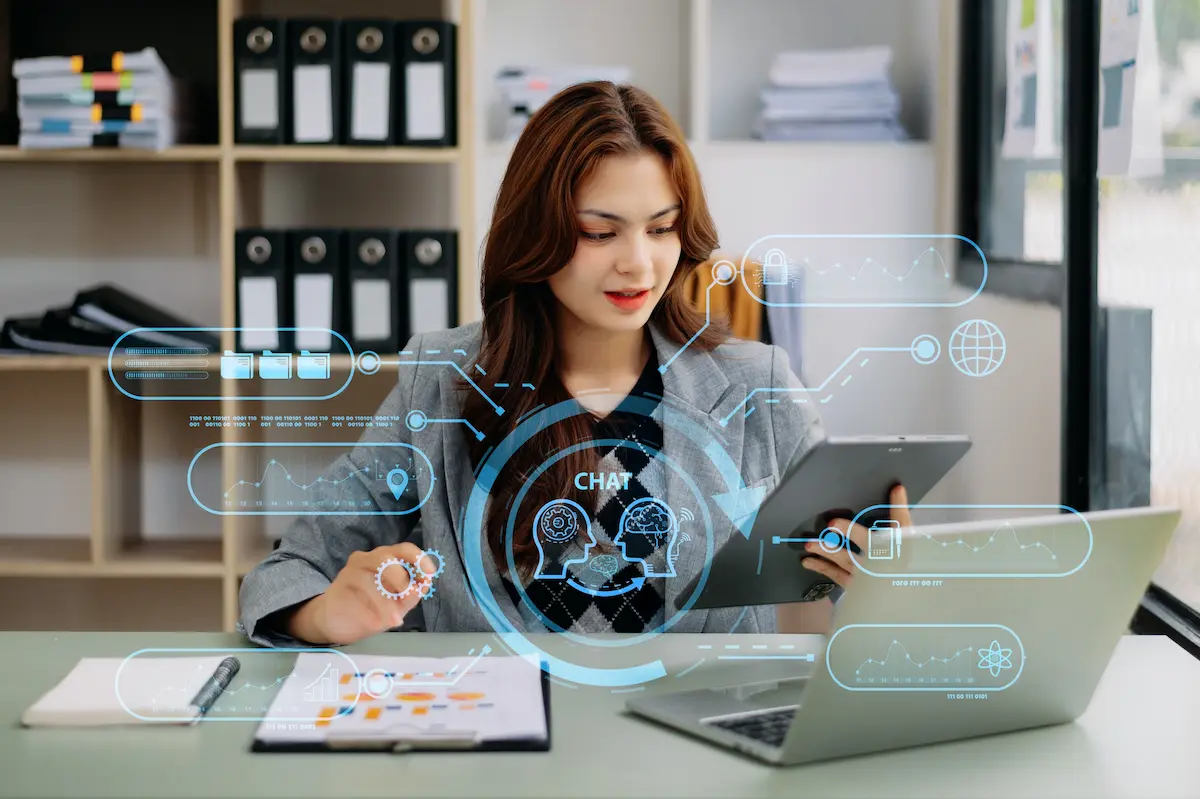
What is Artificial Intelligence Analytics?
AI analytics automates the traditionally labor-intensive and time-consuming process of data analysis by leveraging modern artificial intelligence and machine learning technologies.
Beyond just structured data, AI algorithms are increasingly capable of analyzing unstructured data through methods like natural language processing (NLP), speech analytics transcription, neural networks, computer vision for image and video analysis, and deep learning.
(Read our blog post "Emerging Software Development Technologies" to know everything about the newest trends)
In other words, AI can analyze more than just numerical data. It can evaluate the effectiveness of marketing strategies on various social media platforms, understand customer preferences, and identify potential improvements in supply chains based on economic projections. AI can provide valuable insights to model market or production strategies, which can then be visualized in virtual reality (VR) for detailed analysis.
To understand the applications of this technology, it's essential to recognize its usefulness. According to MIT BTech engineer Shruti Somankar, there are four pillars of data analytics: Descriptive, predictive, diagnostic, and predictive insights. Companies need to address these pillars to achieve a solid analysis of their businesses. Traditionally, this involved large offices and multiple working groups. However, in 2024, these methods are often insufficient. Experts across various industries are increasingly turning to AI to solve their problems, a grand majority are involved with decision-making regarding their businesses and their customer needs.
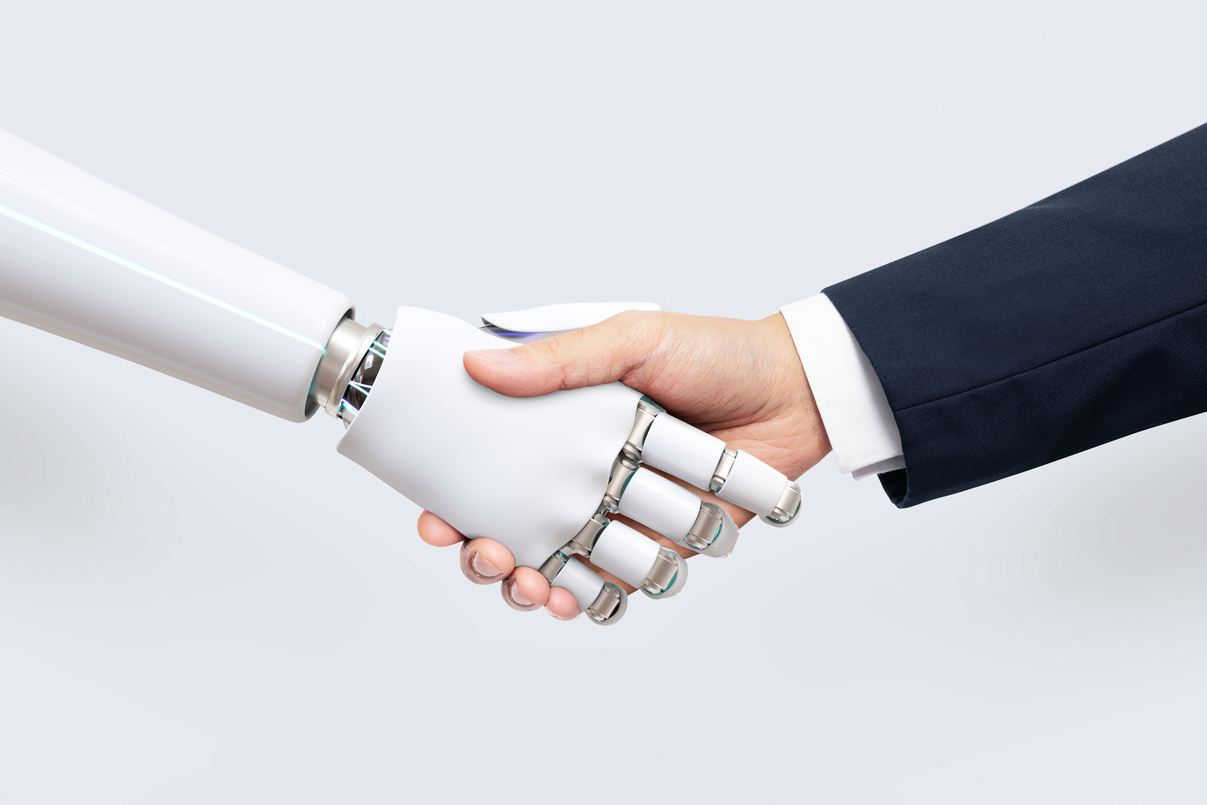
Real-World Applications and Benefits of AI Analytics
Industries worldwide are integrating AI analytics into their operations to improve decision-making and operational efficiency. From predicting market trends and optimizing supply chains to enhancing customer engagement and refining product development, AI offers unprecedented capabilities.
For instance, in healthcare, AI-powered tools are revolutionizing patient care through real-time monitoring and personalized treatment recommendations, while in retail, AI is optimizing inventory management and customer service.
The benefits are manifold: AI analytics could enhance productivity and profitability and facilitate innovation and competitiveness. However, more research and development are still needed to integrate these models fully into such pivotal elements of our society.
Let's dive deeper into the analysis:
Importance of Artificial Intelligence Analytics: The challenge of decision-making in business and a path to growth
Executives and managers face increasing pressure to make sound decisions in the workplace, especially when it comes to customer-related choices. According to research by Oracle and Seth Stephens-Davidowitz, 85 percent of over 14,000 employees and business leaders reported experiencing stress due to the difficult decisions they must make almost daily, many of which are customer-centric. This stress is a symptom of a larger issue: poor business decisions, including those affecting customers, account for at least 3 percent of lost profits, as concluded by a 2018 Gartner study.
The study also found that 61 percent of business and finance executives noted an increase in operational decisions driven by factors such as digitization and mergers and acquisitions (M&A), often impacting customer relations. This trend is not new; decision-making systems that were functional 20 years ago have been outpaced by new ones driven by different variables.
The number of choices we face is multiplying, and more data does not necessarily help. Why does this happen? The key word is "overload." Humans are not super jugglers. We might manage three tasks simultaneously, but handling seven, nine, 16, or 200 becomes impossible.
Stephens-Davidowitz's study reports that 86 percent of subjects said the rapid increase in data volume complicates their personal and professional decisions, including customer-related ones. Additionally, 29 percent felt overwhelmed by it, and more than a third do not trust the sources they use.
The problem is not the amount or sources of data but how we filter, interpret, and rank it. This is where AI becomes invaluable. Just as ENIAC revolutionized computing in 1946, AI technology can drive better decisions faster than any human, including those involving customers. It acts like a superpowered data analyst available through a computer, a smartphone, or even voice-controlled operations. Decision-making is not only crucial for making our lives easier and healthier, but it can also propel our businesses to a new level of competitiveness, and customer satisfaction.
PwC calculates that “AI could contribute up to USD 15.7 trillion to the global economy in 2030, more than the current output of China and India combined.” Of this, PwC estimates that “USD 6.6 trillion is likely to come from increased productivity, and USD 9.1 trillion is likely to come from consumption-side effects.” Much of it is related to analytics, including productivity and customer analytics.
In October last year, Mark Purdy, managing director of Purdy & Associates, and Mark Williams, a senior research scientist at the Institute of Human and Machine Cognition in Florida, published an article in the Harvard Business Review outlining three main ways AI analytics can improve decision-making:
1. Real-Time Monitoring and Enhanced Forecasting of Business Activities
With increasingly fine-grained data from technological tracking of supply chains, firms can now understand where their raw materials and inputs come from, who produced or supplied them, and whether these inputs have been produced and sourced in an environmentally friendly and ethical manner.
Consider the case of South American meat exports to Europe. This activity has been a significant driver of land-use change in Brazil, Paraguay, and Argentina, where the European Union is a strong and preferred importer. However, by December 30th, EU restrictions will prevent any meat business from exporting meat that has caused deforestation or lacks traceability.
AI analysis of satellite imagery can detect unforeseen changes in forest land use in real time, sending alerts to EU inspectors or stock production investors. This technology can also identify abrupt shifts in the forest canopy, providing immediate notifications to managers about potential deforestation risks and allowing them to take preventive measures.
Machine learning and deep learning AI analytics tools have immense algorithms that can identify the colors of satellite images to identify land-use covers such as forests, soy cultivation, or pastures. These tools are evolving rapidly, and complex data cross-referencing can now be resolved in just a few seconds.
Moreover, AI analysis enables stock producers to implement sustainable feeding programs that rotate pastures to minimize soil impact and optimize export products. These tools can recommend schedules and routes for pasture rotation and more, ensuring more sustainable and efficient agricultural practices.
These are just some of the variety of applications that machine and deep learning AI could be used for.
IBM lists many capabilities of these emerging technologies. For analytics, they highlight the orchestration pipelines, a unified platform that provides teams with a consistent set of tools for data analytics, data science, and machine learning, supporting a wide variety of algorithms, including neural networks for advanced predictive analytics. These artificial networks try to copy the synaptic connexions in our brains. It involves millions of information processing in just seconds.
IBM also suggests considering automated machine learning, decision optimization, automated development, and synthetic data generator tools. With this, we just need to give the AI analytics tool access to the information we need to process, and the algorithm will interpret the data, synthesize it, and suggest the best paths to choose to get to a specific objective like the best place or time to invest, the weak points in a productive or commercial strategy, identify the needs of our customers, or even analyze the competition.
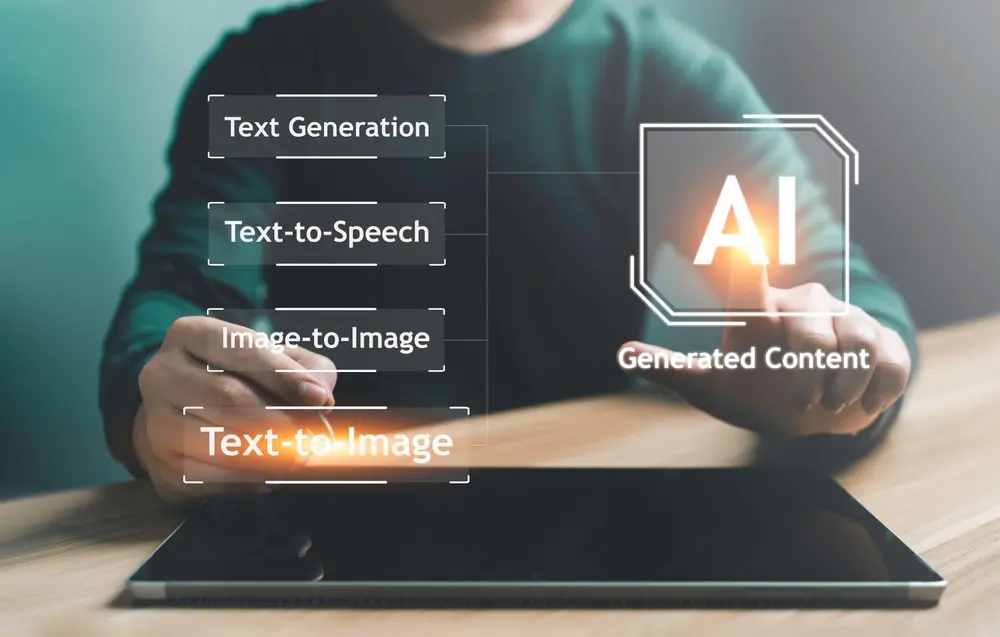
2. Virtual Role-Play for Training Employees in Realistic Business Situations
From the simplest to the most complex applications, AI analytics offers a variety of options for businesses across multiple industries. One of the most innovative uses is virtual role-play for training in fields such as healthcare, automotive, aeronautics, retail, construction, and education, driven by advanced algorithms.
These tools allow junior employees to practice interacting with carefully designed virtual customers or enable biology students to analyze the behavior of different species without leaving their school or university, thanks to sophisticated algorithms. An example of this technology is zSpace Inspire, which uses augmented reality (AR) to dissect animals, observe their inner composition, analyze human anatomy, or see how an internal combustion engine functions.
zSpace Inspire is an immersive educational platform combining AR and virtual reality (VR) technologies to revolutionize learning. It features a specialized workstation with a high-resolution AR/VR display and a stylus for interaction. The system offers interactive content across subjects like science, technology, engineering, and mathematics, allowing students to engage with virtual objects, conduct experiments, and explore complex concepts hands-on. This approach not only enhances understanding but also fosters critical thinking and collaboration among students, facilitated by advanced algorithms.
As exciting as this technology is for education, virtual reality has many other applications. Many large companies use it to place employees in different scenarios. For example, Walmart has used VR tools to train workers at several levels since 2017, covering situations from a robbery to Black Friday. At least one million employees have learned how to respond to various situations in different roles within the company by using Oculus VR headsets for training, leveraging sophisticated algorithms to simulate real-world experiences.
3. New Generative AI Tools Serving as Advisors and Virtual "Sounding Boards" for Decision-Makers
Imagine an advisor who remembers everything, is fully focused, and never gets tired. This is what generative AI offers. The most common example is ChatGPT, a famous chatbot search tool that uses “large language models” (LLMs)—multi-layer neural networks trained on large amounts of data to simulate human conversation. In just two years, similar tools have evolved and diversified, including Google’s Gemini, Microsoft Bing, Chatsonic, Github Copilot, and others. Startups and AI companies have also developed technology tailored for specific industries, with healthcare being a particularly interesting case.
Last year saw a surge of interest in generative AI applications in healthcare. LLMs have already shown their utility in passing exams like the United States Medical Licensing Examination, generating research articles, and interpreting data from electronic medical records (EMRs).
According to an article published this year by NPJ Digital Medicine, generative AI models trained on EMR data are particularly relevant to patient care. These LLMs can analyze notes, lab results, and billing codes, offering improved predictive accuracy, streamlined model development, and cost-effective deployment.
In April, Microsoft announced a partnership with Epic EHR to integrate its OpenAI service, potentially automating responses to frequently encountered or labor-intensive patient messages. Additionally, Oracle Cerner has incorporated generative AI into its EHR system.
In November 2023, Oracle Cerner unveiled the Oracle Clinical Digital Assistant, a multimodal tool combining voice and screen interfaces that actively participates in appointments by automating note-taking and suggesting actions like medication orders, lab tests, and follow-up appointments.
Despite these advancements, challenges remain. Researchers like Stanford Computer Science PhD student Michael Wornow highlight the limits AI development still needs to resolve for its integration into the health industry. Wornow and his peers developed an evaluation framework for generative AI in healthcare, proposing a comprehensive approach to its implementation. This approach should include clear leadership in development, incentives for adoption, and ongoing regulatory oversight.
Effective leadership is crucial for ongoing model development, validation, and adoption. Currently, various entities such as startups, research groups, and academic healthcare systems are creating generative AI models. However, a central leadership body is needed to guide practical implementation.
This leadership should establish performance guidelines for models, promote data sharing, identify optimal healthcare settings for clinical trials using generative AI, and address the specific needs of different healthcare environments. Ideally, this leadership would involve stakeholders like physicians, healthcare administrators, developers, and investors, possibly coordinated by a sub-committee within the FDA. Ongoing regulation is necessary to balance the interests of developers, healthcare systems, payers, and patients.
Generative AI tools are being developed to address particular situations, such as administrative processes, legal analysis, and academic purposes. Each tool has its strengths and weaknesses, but polished models are only a matter of time.
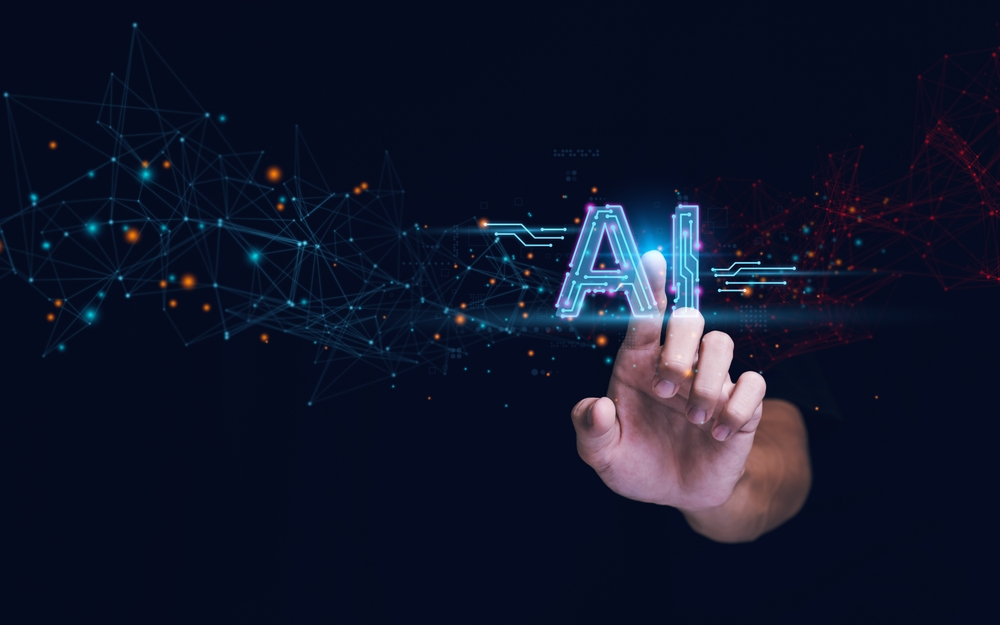
Choosing the right AI analytics tools
Every blog or expert who suggests which AI tool is the most accurate emphasizes the importance of clarity as the first step. This means defining the specific processes we want to replace with AI and projecting the expected outcomes in the short and long term.
If our goal is to respond to emails as quickly and accurately as possible, we will likely need a content generator. This type of generative AI can produce text, images, and other content based on the data we provide, meaning it must be trained accordingly. However, if we aim to analyze financial market dynamics, we will probably need a different type of technology that can process real-time reports and provide insights for an investment strategy.
Additionally, we must consider the compatibility of these new systems with our current infrastructure. As The Economist highlighted in an article a few years ago, not considering the adaptability of our business to AI tools could delay the entire productive system. It is crucial to prepare for the changes this technology may bring, such as shifts in job roles, fact-checking strategies, and workflow dynamics. Employees and managers, especially in high-pressure jobs, must feel comfortable with this technology to implement it effectively.
Another relevant point is the potential return on investment. We should ask ourselves: How much profit could this AI tool bring to our business? How long will it take to achieve this return? Does our initial investment, along with employee training and maintenance costs, justify the benefits?
Finally, and most importantly, we must consider security and compliance. How can we ensure that our data won’t end up in the wrong hands? Every AI tool should specify that it adheres to data protection regulations relevant to our industry and laws.
With these considerations in mind, it's just a matter of searching through the thousands of AI options that appear every day for every possible business need.
Conclusion
As AI technologies continue to evolve, their potential to drive economic growth and foster transformative changes across industries becomes increasingly evident. By embracing AI analytics, businesses can unlock new opportunities for growth and efficiency, positioning themselves at the forefront of the digital age.