Business efficiency is every company’s dream. Every enterprise tries to be as productive and efficient as possible, from food businesses to tech companies. The pursuit of productivity has been the dream for companies and economic policymakers. Throughout the years, everyone has been trying to figure out how to produce more with less. Artificial intelligence has been a lingering promise, offering the tantalizing prospect of automating processes, monitoring for errors, and much more; which can lead to significant cost savings by reducing labor costs and minimizing errors.
The last time productivity boomed was in the 1990s, caused by the widespread adoption of a shiny new tool that revolutionized the workplace: computers. The advent of computers unleashed unprecedented possibilities for efficiency gains, laying the foundation for subsequent advancements in technology-driven productivity.
Will this decade go down in history as the new productivity-revolutionized one? Research shows that AI can help less experienced workers enhance their productivity more quickly. Is AI worth the risks it entails, or can we harness its benefits while mitigating them?
How Companies are Adopting AI
The premise now seems to be: If there is an annoying task, there’s an AI for that. Well, AI can certainly be useful when used in reiterative tasks or when a thorough eye is needed. A study done by IBM on companies around the globe found that “about 42% of enterprise-scale organizations (over 1,000 employees) surveyed have AI actively in use in their businesses”. When we look at the list of operations where companies have already started deploying the use of AI, we can confirm that:
Automation of IT processes (33%)
Security and threat detection (26%)
AI monitoring or governance (25%)
Business analytics or intelligence (24%)
Automating processing, understanding, and flow of documents (24%)
Automating customer or employee self-service answers and actions (23%)
Automation of business processes (22%)
Automation of network processes (22%)
Digital labor (22%)
Marketing and sales (22%)
Fraud detection (22%)
Search and knowledge discovery (21%)
Human resources and talent acquisition (19%)
Financial planning and analysis (18%)
Supply chain intelligence (18%)
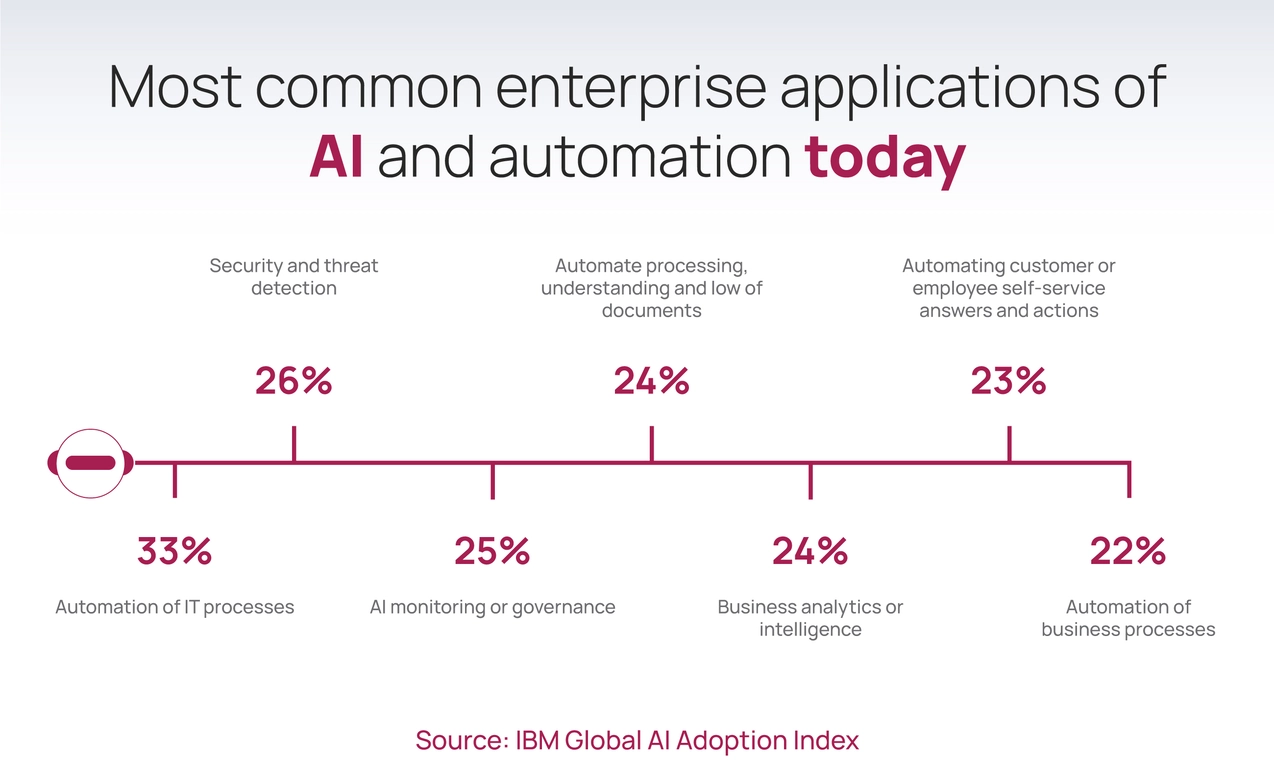
There are quite a few examples of successful AI integration. With over 20 years of experience in the Technology industry, we are no strangers to technological advancements and innovation. We have started testing controlled deployments of the technology in our developments, such as Cosmic Latte’s recent updates.
We recently launched a new version of Cosmic Latte that has been integrated with OpenAI and is currently being tested by selected users. This latest version allows rapid, personalized workplan generation for 360 evaluations using an LLMS (Large Language Model Service) based on Open AI 3.5 and Open AI 4.0. This advancement benefits managers and team leads who are in charge of large teams of engineers by automatically gathering work plans for 360 evaluations.
By automating the collection of work plans, managers can more effectively identify and understand the goals and objectives for their engineers, enabling them to provide targeted support and guidance.
Ultimately, this facilitates engineers' personal and professional growth, fostering an environment conducive to their development and success within the organization.
Another example is customer-care chatbots, which have been found to reduce customer care costs significantly while increasing brand proximity due to their ability to provide immediate responses around the clock. A study within a Brazilian commercial bank highlighted that AI chatbots improved service efficiency, evidenced by 181 million interactions and 7.6 million attendances in 2020. They reduced queues for call centers, enabling human attendants to handle more complex tasks.
In line with this trend, Jalasoft also deployed a beta version of a chatbot named Ignite Mate, designed to assist users in getting started with our blockchain-based solution, Jala Ignite. This initiative aimed to streamline the onboarding process for users, providing them with immediate support and guidance whenever they needed assistance.
Artificial Intelligence’s Double-Edge Sword
If AI can be such a wonderful tool, why isn’t everyone using it? While some companies have begun to embrace AI, most are still reluctant to actively deploy the technology in their business. A significant portion of the companies surveyed by IBM, about 40%, remains in the Exploration and Experimentation Phases.
At Jalasoft, we are acutely aware of the potential risks associated with AI integration into software development processes. As a result, our developers do not use AI tools in their daily work unless explicitly authorized by the client and under conditions specified by the client. Instead, we reserve AI experimentation exclusively for internal projects within our company. This approach ensures that we prioritize client confidentiality and security while maintaining a commitment to innovation and exploration within our own operations.
The reality is that AI adoption is still complicated. With new advancements being shown to the world practically every day, the technology is still in the development stages, and there are many aspects where there is still a need for improvement. To explore potential risks and benefits, we have created a special unit within our Research and Development department, which is currently researching and testing ways to integrate AI into our tools, but also investigating possible downsides of the technology’s integration and ways to avoid them. One of the main concerns we share with the world is in respect of Data Privacy and intellectual property protection.
When queried about the reasons for their reluctance to deploy traditional AI, companies cited several key barriers that included limited AI skills and expertise (33%), challenges related to data complexity (25%), and ethical concerns (23%).
The case gets more complex with the use of Generative AI, with its biggest inhibitors, according to IT professionals at surveyed organizations, being Data privacy (57%) and trust and transparency (43%). According to research from Cyberhaven, a data security firm, 2.3% of employees inserted confidential company data into ChatGPT in its first three months of use, with sensitive data making up 11% of what employees paste into the Generative AI chatbot.
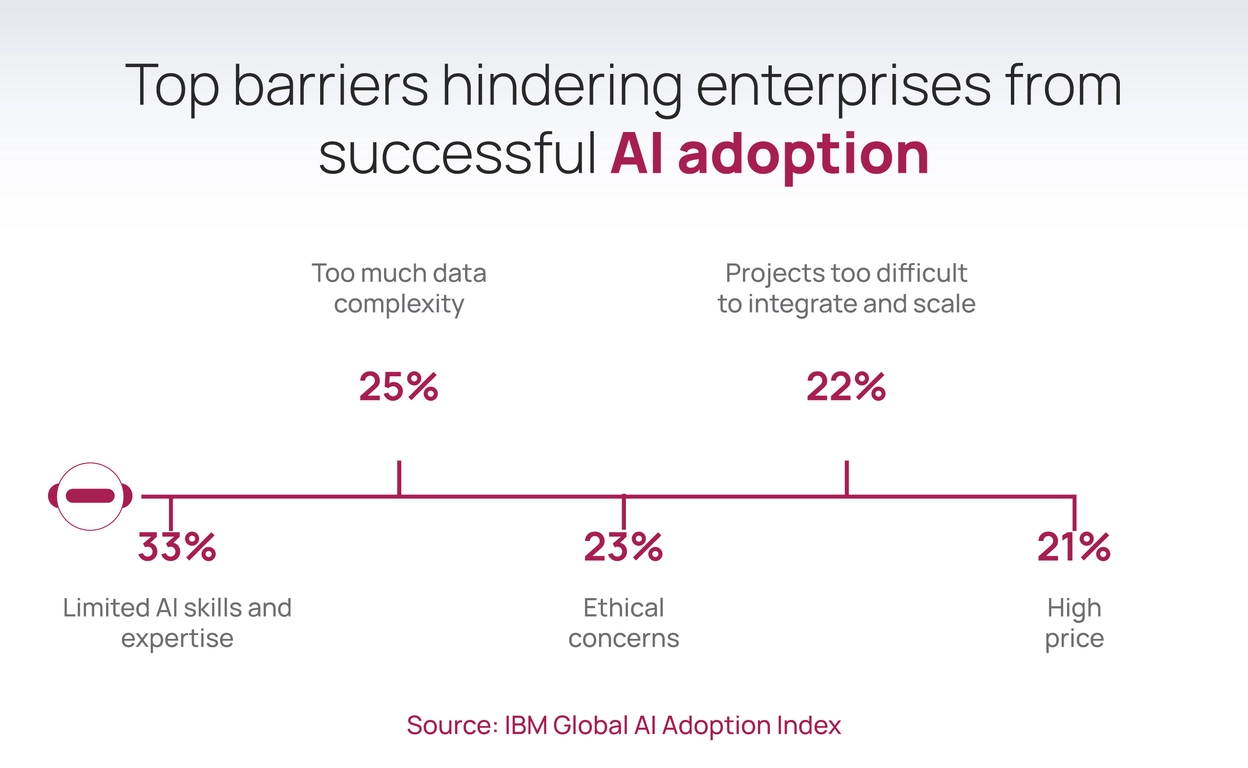
Data privacy regulations such as the GDPR (General Data Protection Regulation) in Europe and the CCPA (California Consumer Privacy Act) in the United States have heightened awareness about the importance of protecting individuals' privacy rights. AI systems often rely on vast amounts of personal data to train their algorithms and make predictions. However, the collection, storage, and analysis of sensitive data raise concerns about individuals' privacy rights and the potential for unauthorized access or misuse of personal information.
Non-compliance with data privacy regulations can result in significant financial penalties and reputational damage for companies. The GDPR, for example, imposes fines of up to €20 million or 4% of annual global turnover, whichever is higher, for violations. Similarly, the CCPA allows for statutory damages of up to $750 per consumer per incident, with the potential for class-action lawsuits.
Aside from regulations, there are other privacy and security concerns. Although AI can be used as a security shield, for instance, to identify patterns and anomalies that might indicate a potential cyberattack, or to scan networks and systems for vulnerabilities; it can be a double-edged sword. As mentioned before, AI requires large volumes of data to function effectively, even if used as a security solution. If the data is not properly secured, using an open-source AI could lead to a potential data privacy risk, there could be a breach. Human error in handling data can further exacerbate these risks. Why not train our own AIs then? Well, that would require not only skills and expertise (which 33% of companies said they lack) but a whole lot of work.
Breaches can erode customer trust and loyalty, leading to loss of business and damage to brand reputation. In industries such as healthcare, finance, and technology, where sensitive data is a valuable asset, unauthorized access or disclosure of proprietary information can lead to competitive disadvantage and loss of market share.
This gets more complicated when dealing with intellectual property. OpenAI has faced several lawsuits from authors who claim that their copyrighted works were used without permission to train OpenAI's language models. These lawsuits argue that the AI's outputs, such as text completions and summaries, are derivative works that infringe on the original copyrights. This situation highlights the complex legal challenges in determining whether AI-generated content using training data derived from copyrighted materials constitutes fair use or copyright infringement. This could affect many lines of business, from marketing to software development.
Ethical considerations
Furthermore, the ethical implications of AI extend to broader societal issues, such as autonomy, accountability, and control. The root of this issue is that deep-learning programs are trained by rearranging their digital innards in response to patterns they spot in the data they are processing. They are created based on how neuroscientists believe our brains learn, by changing within themselves the strengths of the connections between bits of computer code that are designed to behave like neurons. This makes it impossible for anyone, not even the designer of that neural network, to know how exactly the machine is doing what it does.
Now if we let AI algorithms make decisions, in areas like medical decisions, law enforcement, and such, we’d be trusting people’s lives to pieces of equipment whose operation no one truly understands.
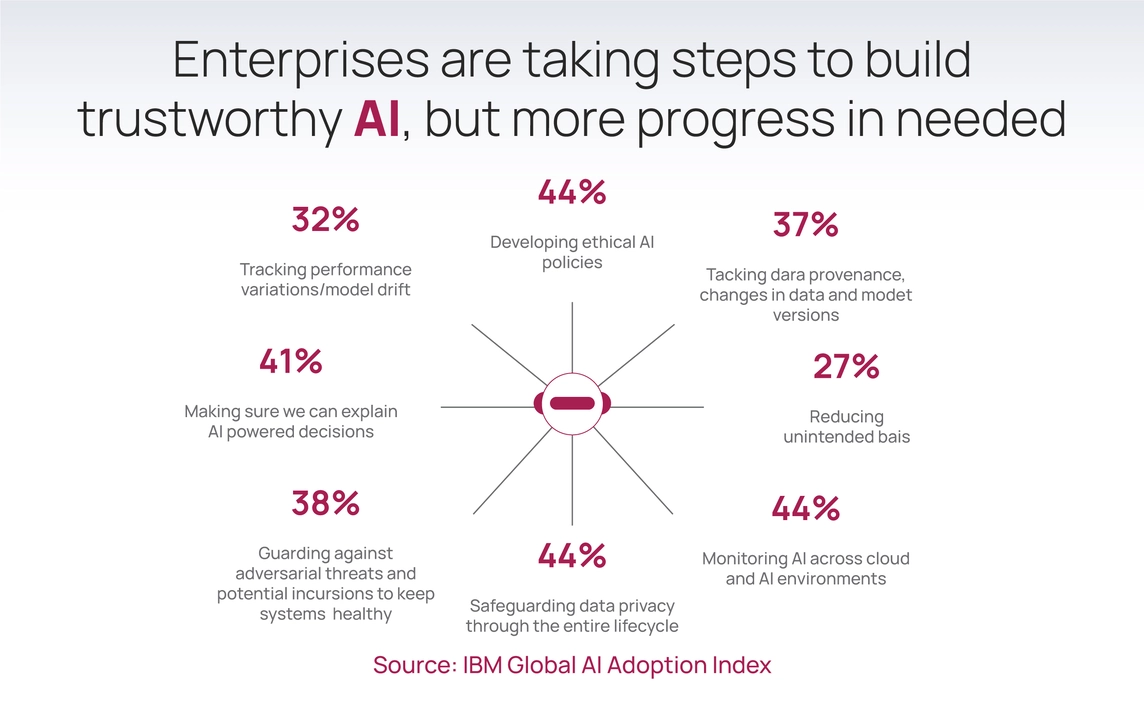
Yes, AI-powered recommendation systems in areas such as finance, healthcare, and education can enhance productivity, reduce time, and make things more efficient. But what is the cost? They have the potential to perpetuate existing biases and exacerbate social inequalities if not carefully designed and monitored. As AI systems become more sophisticated and autonomous, questions arise about who bears responsibility for their actions and how to ensure they align with ethical principles and values.
At Jalasoft we know that as we enter a world where AI complements our abilities daily, human-centric skills will be significantly more important. That's why we prioritize the training of our engineers in these critical areas from the earliest stages of their education.
We develop and apply policies for responsible use, including restrictions on the generation of harmful or deceptive content. Furthermore, we collaborate with regulatory bodies and standard organizations to promote the safe and ethical use of AI.
Companies should conduct ethical impact assessments, which involve evaluating the potential ethical implications and societal impacts of AI systems throughout their lifecycle. These assessments help identify and mitigate risks such as algorithmic bias and unintended consequences before they manifest in real-world applications.
In addition to ethical impact assessments, companies should also prioritize transparency and accountability in their AI initiatives. Always provide clear explanations of how AI systems work, including their data sources, algorithms, and decision-making processes, as well as the potential risks and limitations associated with their use.
Final takeaways by Jalasoft
The promise of AI is immense; with new developments and corrections deployed almost regularly, it seems like the sky is the limit. However, we must be cautious and refrain from jumping in without prior assessment, the consequences could be disastrous for our companies, and for the world. Here are some final takeaways and recommendations:
Before allowing any implementation of AI, take a moment to carefully assess the advantages and dangers. Always be clear and transparent with users about the tests being made.
Always communicate effectively and transparently the use of AI, sources, and possible risks of use.
If possible, invest in an AI-specialized team that can assess the opportunities and implications of the technology in your business field.
It might make sense to invest in training an AI on specialized data sources for very specific purposes.
When hiring a new company, review its policies around AI and its approach to compliance.